On June 1, 2022, an original article was published online by Prof. Xiulan Zhang’s team in Journal of Clinical Investigation (JCI, IF=19.3) on predicting the incidence and progression of glaucoma based on color fundus photos using artificial intelligence (AI). This is the 11th publication focusing on glaucoma AI after a series of works published in NPJ Digital Medicine,Ophthalmology, and Medical Image Analysis.
The study is composed of two parts: the onset prediction and progression prediction of glaucoma.
Incidence prediction aims to evaluate the risk of onset of glaucomatous optic neuropathy in the next 3—5 years based on the baseline color fundus photographs (CFPs). First, each CFP was labeled by three physicians into glaucoma and non-glaucoma. The chronological order of the pictures was randomly disrupted during labelling.
Then the follow-up data of the same eye were divided into two categories: Class I was non-glaucoma at baseline and became glaucomatous during follow-up (positive samples); Class II was non-glaucoma at baseline and remained non-glaucoma at the subsequent follow-up visits (negative samples).
Based on the above data, an incidence prediction model was developed to predict the probability of glaucoma onset based on baseline CFPs. The incidence prediction model consists of two parts: a segmentation module (Unet) and a diagnostic module (ResNet) (Figure 1), which were trained based on the dataset of 10,357 eyes (112 positive samples and 10,246 negative samples). Validation was performed in two external population cohorts with 1674 eyes and the AUC values were 0.89 and 0.88.
Progression prediction refers to the assessment of the risk of visual field progression in the next 3–5 years based on the baseline CFPs. All the subjects had paired CFPs and visual field reports. Visual field reports were assessed by three physicians and divided into two groups: with visual field progression (positive samples) and without visual field progression (negative samples). Based on the above data, a progression prediction model was developed to predict the probability of future glaucoma progression based on the baseline CFPs. The progression prediction model also consists of two parts: a segmentation module (Unet) and a predictive module (ResNet) (Figure 1), which were trained based on data from 3003 eyes (327 positive samples and 2676 negative samples). Validation was performed in two external cohorts with 850 eyes, and achieved AUCs of 0.87 and 0.88.
In interpretability analysis, the heatmaps indicated that the AI models predicted the risk mainly based on the optic disc boundary, rim and vessel arcades, which was quitesimilar to clinicians when assessing glaucomatous damage (Figure 2).
This is the first study to create glaucoma prediction models based on baseline data on a large dataset of longitudinal CFPs. The baseline CFPs images were input into the AI models, and the risk of healthy subjects developing glaucoma or visual field progression in glaucoma patients in the next 3—5 years were generated. The highlights of the study are: 1) Developing AI models to predict glaucoma incidence and progression for the first time, and achieving robust predictive performance in the external test sets; 2) the data for glaucoma incidence prediction were collected from the community cohorts, which better reflects the distribution characteristics of glaucoma in the Chinese population, and strengthens the generalization and reliability of AI model; 3) AI model successfully predicts functional change based on structural data, and achieved high predictive accuracy for different subtypes of glaucoma, including POAG and PACG.
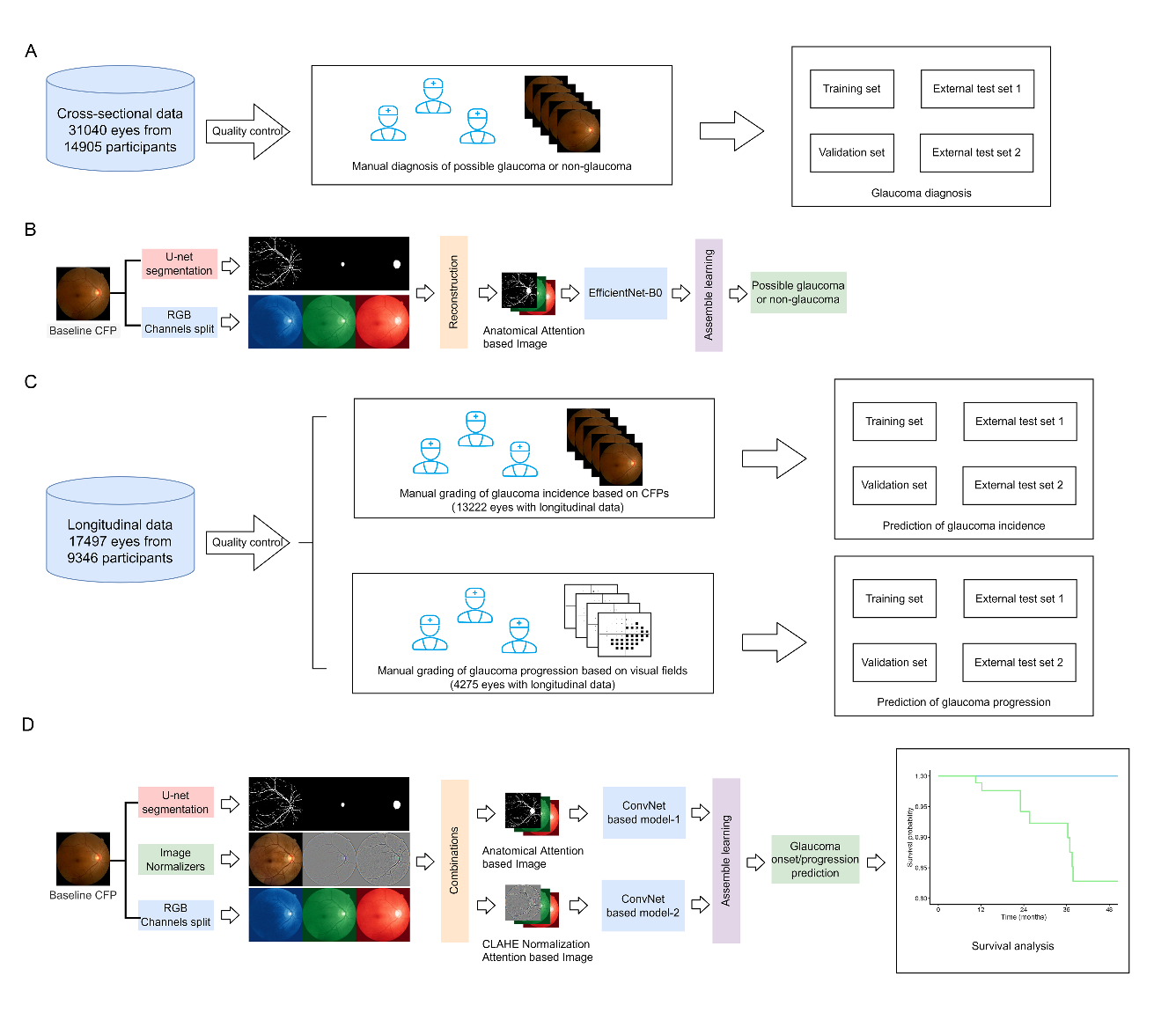
Figure 1. Schematic diagram of the design and validation process of deep learning system for the prediction of glaucoma onset and progression.
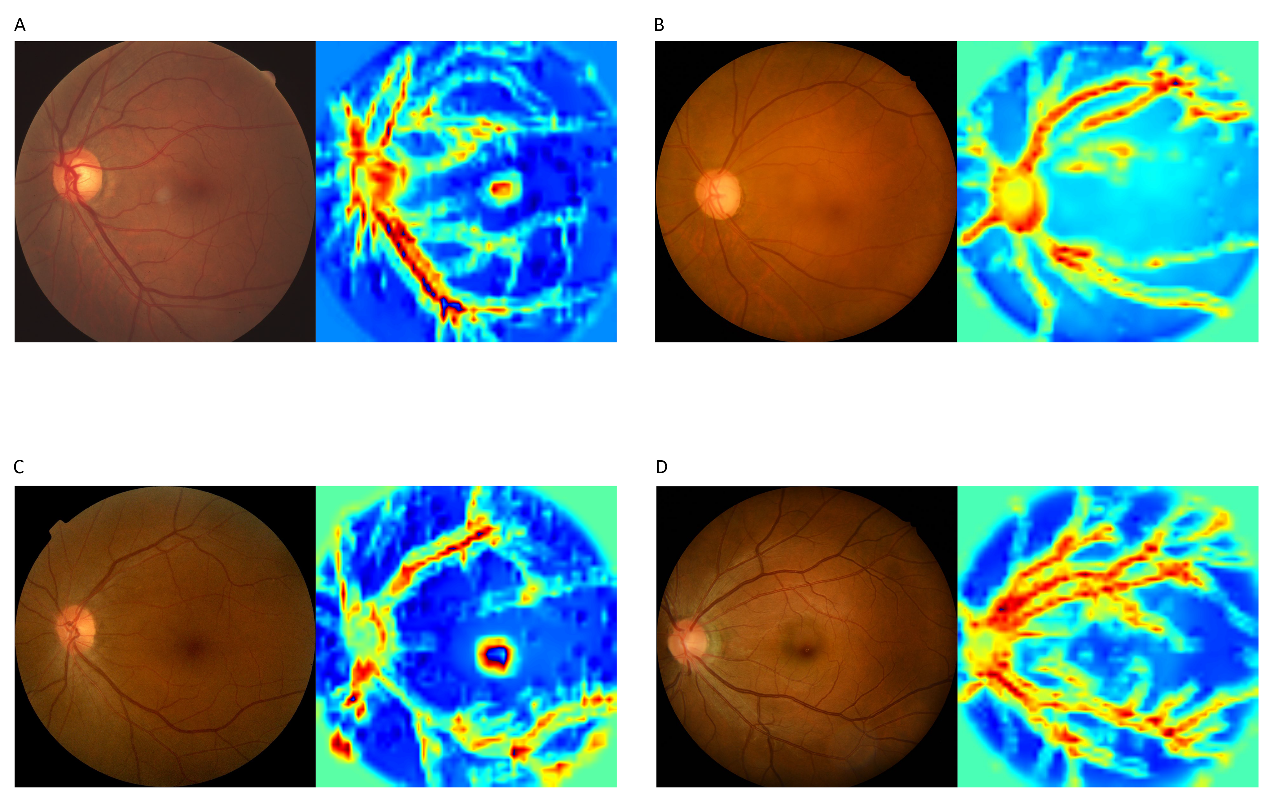
Figure 2. The heatmaps of deep learning model indicated that AI model predicts the onset (A, B) and progression (C, D) mainly focusing on the optic disc rim and vessel arcades, which was very similar to the clinicians.
In recent years, with the support from the Clinical Research Center of Zhongshan Ophthalmic Center, Prof. Xiulan Zhang’s team has performed an in-depth exploration from the construction of glaucoma database, the development of algorithms to the establishment of the most comprehensive intelligent system for glaucoma prevention, screening and diagnosis (iGlaucoma) in the world. Her team had published 16 relevant high-quality articles, among which 10 articles were published in Class 1 medical journals, including NPJ Digital Medicine, Ophthalmology, Medical Image Analysis, IEEE Transactions on Medical Imaging, etc. She had registered nine invention patents (including 2 PCT patents). One patent and one software copyright had been approved. The largest ophthalmic multi-disease data-sharing platform around the world --iChallenge was established by her team (https://ichallenge.grand-challenge.com), which had supported the publication of 292 scientific research papers by April 26, 2022. Moreover, the team had led the development of the first Chinese standard for the quality control of color fundus photography data, Annotation and quality control specifications for fundus color photographs (T/CAQI 166-2020) and established the project at WHO-ITU (No.SG16-TD227/WP2), which contributes to standardizing the emerging development of ophthalmic AI. In the future, her team will further explore the application of AI technology to simplify and unify the glaucoma diagnosis and treatment process, and realize the standardization, digitization and intellectualization of glaucoma diagnosis and treatment.
The first authors of the article were Fei Li (Zhongshan Ophthalmic Center, ranked as the first), Yuandong Su (Sichuan University), Fengbin Lin (Zhongshan Ophthalmic Center) and Zhihuan Li (Macau University of Science and Technology); Xiulan Zhang (Zhongshan Ophthalmic Center, the last corresponding author) and Kang Zhang (Macau University of Science and Technology) were the co-corresponding authors. State Key Laboratory of Ophthalmology, Zhongshan Ophthalmic Center, Sun Yat-sen University, Guangdong Provincial Key Laboratory of Ophthalmology and Visual Science, Guangdong Provincial Clinical Research Center for Ocular Diseases were listed as the first affiliations. A total of 28 researchers from 23 domestic institutions participated in this study.
Link to the article: https://doi.org/10.1172/JCI157968