Antibiotics were one of the most important discoveries in the history of medicine during the 20th century. Antibiotic resistance, however, has become a major concern worldwide due to antibiotic abuse or misuse. As a genetic phenomenon, antibiotic resistance occurs when bacteria evolve in response to selective pressures imposed by antibiotics. Specifically, random mutations can occur at every nucleotide site in antibiotic resistance genes, making the host bacteria more sensitive or more resistant to antibiotics. When antibiotics are misused, the resistant mutants will likely outgrow the sensitive mutants, resulting in the progressive spread and transmission of antibiotic resistance.
Towards understanding this evolutionary process and, ultimately, predicting and controlling the evolution of antibiotic resistance, the research teams led by Prof. Xiaoshu Chen, Prof. Jian-Rong Yang, and Prof. Guobao Tian from Zhongshan School of Medicine at Sun Yat-sen University, conducted a collaborative study. They constructed an E. coli mutant library containing all single nucleotide mutants of blaCTX-M (an extended-spectrum β-lactamase resistance gene which is highly prevalent in hospital) and combined high-throughput experiments with deep learning models to predict the evolution of blaCTX-M. Their results were recently published as "Prediction of Antibiotic Resistance Evolution by Growth Measurement of All Proximal Mutants of Beta-Lactamase" in Molecular Biology and Evolution (IF16.240), a top-tiered journal in the field of Evolutionary Biology.
The experimental part of the study consists of four major steps. First, doped oligonucleotide was synthesized with a mutation rate of 3% per site, which was then concatenated with the remaining wild-type regions via multiple rounds of fusion PCR to generate the mutant library. During this process, a random 20 bp barcode was also attached as an index of the genotypes. Second, the blaCTX-M mutant library was bulk-transformed and integrated into the attHK022 site of E. coli MG1655 as a single copy. Third, the correspondence between blaCTX-M genotype and the 20bp barcode was determined by PacBio-CCS sequencing. Fourth, pooled competitive growth of all the mutants was followed by regular high throughput sequencing of the barcodes to determine the relative growth of the mutants in the presence of antibiotics (i.e., the antibiotic resistance). Supporting the validity of this method, mutations that are proximal to the active center of CTX-M, such as P167, L169, E166, are associated with significantly greater changes in relative growth, which is also in agreement with individually measured MICs (Figure 1).
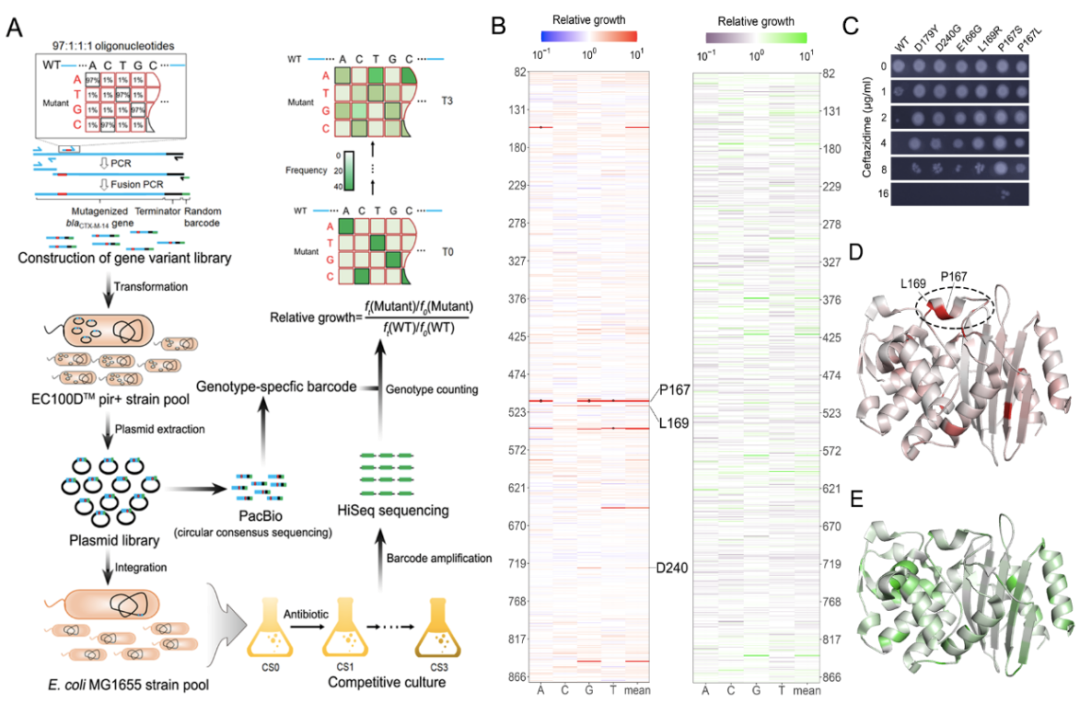
Figure 1. Fitness landscape of antibiotic drug resistance gene
Based on the experimental data, the research team constructed two neural network models for prospective (PEARP) and retrospective (PEARR) prediction of CTX-M's evolution. Here, PEARP is able to predict whether a new mutant is resistant, with an AUC of 0.928. There was a significant enrichment among clinical isolates of the resistant genotypes predicted by PEARP (including single-, double-, triple-, and quadruple-mutated genotypes), suggesting that the prediction of PEARP has clinical relevance. The PEARR can be used to retrospectively predict the evolutionary trajectory of CTX-M, which appeared to be highly consistent with the trajectories revealed by independent drug susceptibility experiments under the SSWM (Strong-Selection-Weak-Mutation) model (Figure 2).
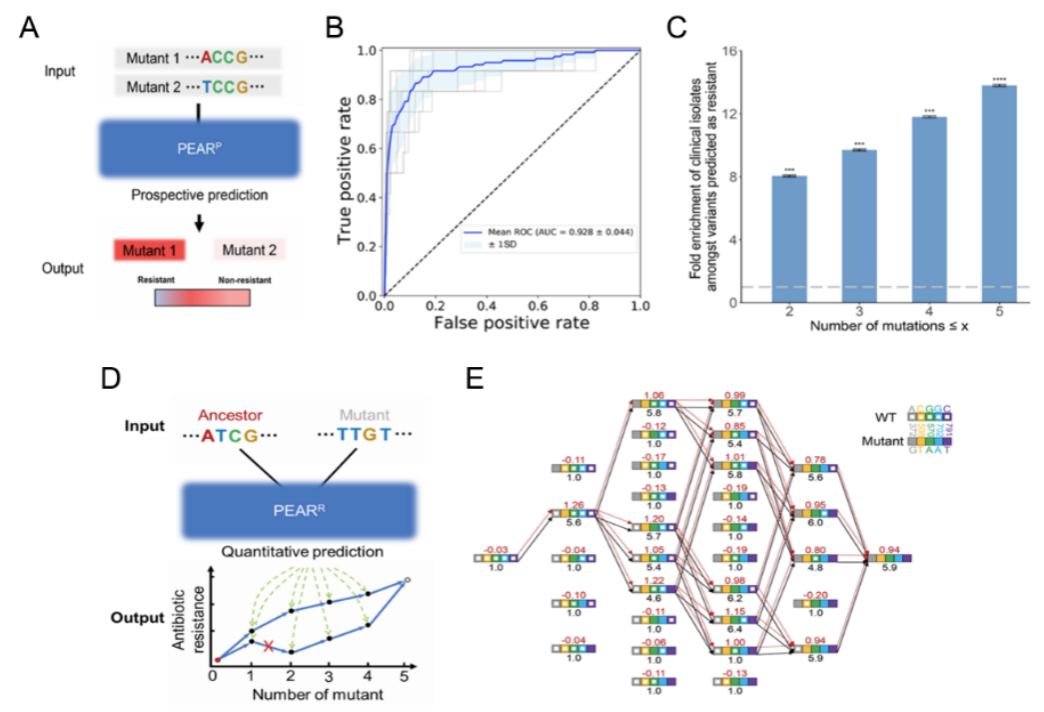
Figure 2. Retrospective and prospective prediction model for antibiotic drug resistance gene evolution
In summary, the high-throughput platform developed can be used to predict the evolution of antibiotic resistance genes. These results have important implications for the proper use of current antibiotics, the design of new antibiotics, as well as, for the prevention and control of clinical antibiotic resistance.
Siyuan Feng (associate researcher), Zhuoxing Wu (PhD candidate), and Wanfei Liang (PhD candidate) from Zhongshan School of Medicine at Sun Yat-sen University are the co-first authors. Prof. Xiaoshu Chen, Prof. Jian-rong Yang and Prof. Guobao Tian from Zhongshan School of Medicine at Sun Yat-sen University are co-corresponding authors.
This work was supported by the National Natural Science Foundation of China (grant number 81830103, 82061128001, 81722030, 32122022, 31871320, 31771406, 82002173), Guangdong Natural Science Foundation (grant number 2017A030306012), National Key Research and Development Program (grant number 2017ZX10302301), the National Special Research Program of China for Important Infectious Diseases (grant number 2018ZX10302103), the National Key R&D Program of China (grant number 2017YFA0103504, 2021YFF1200904), Project of high-level health teams of Zhuhai at 2018 (The Innovation Team for Antimicrobial Resistance and Clinical Infection), and 111 Project (grant number B12003)
Link to the paper: https://doi.org/10.1093/molbev/msac086