Drug discovery and development have huge potential benefits for the pharmaceutical industry and patients. The identification of wet-lab experiment technology is expensive and time-consuming. Therefore, the use of artificial intelligence methods to identify potential drugs can significantly reduce costs and greatly shorten the drug development process. Molecular representation is the basis for reliable quantitative structure–activity/property relationship. However, molecular representation still faces several urgent problems, such as the polysemy of substructures and unsmooth information flow between atomic groups.
Recently, the team of Prof. Calvin Yu-Chian Chen from the School of Intelligent Systems Engineering, Sun Yat-sen University, published a research paper online entitled "Mol2Context-vec: learning molecular representation from context awareness for drug discovery" in the internationally renowned journal Briefings in Bioinformatics. This work proposed a novel deep contextualized bidirectional long short-term memory (Bi-LSTM) architecture, Mol2Context-vec, which can integrate different levels of internal states to bring abundant molecular structure information. And the obtained molecular context representation can capture the interactions between any atomic groups, especially a pair of atomic groups that are topologically distant.
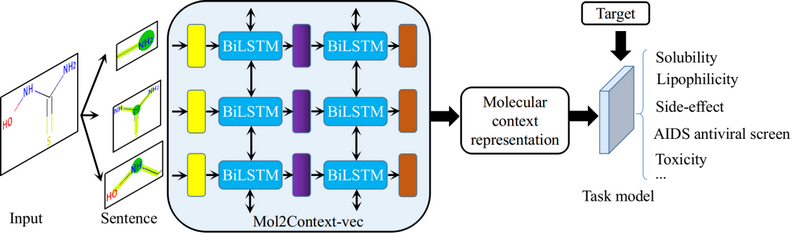
Fig 1. Overview of network architecture and usage steps of Mol2Context-vec.
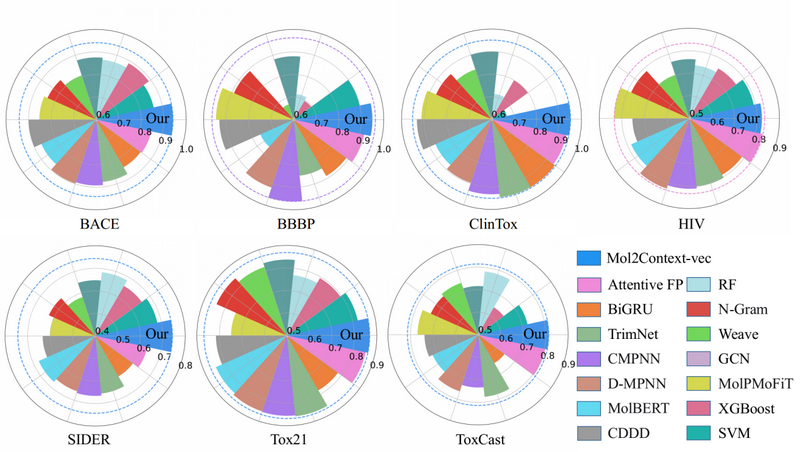
Fig 2. Compared with 14 baseline methods, the predictive performance on datasets related to biological activity and physiology
Mol2context-vec achieves state-of-the-art performance on multiple biochemical benchmark datasets, which proves the competitiveness of this work in promoting molecular representation learning. The model also provides easy to interpret model results, which will improve researchers' potential factors understanding of molecular activity and toxicity.
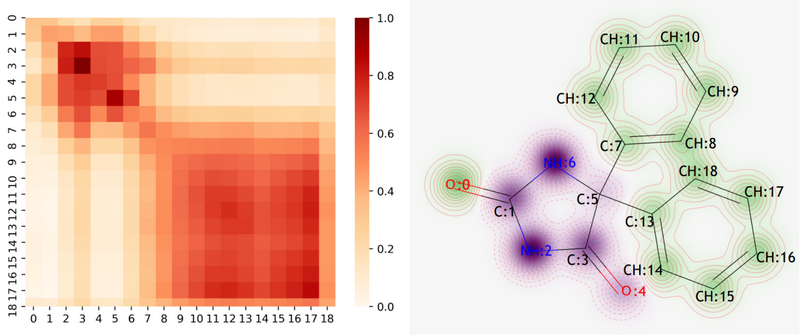
Fig 3. Chemical intuitive explanation of Mol2Context-vec on phenytoin. (a) The heat map of Phenytoin’s atomic similarity matrix. (b) The contribution visualization of each atom in the phenytoin molecular structure to the solubility.
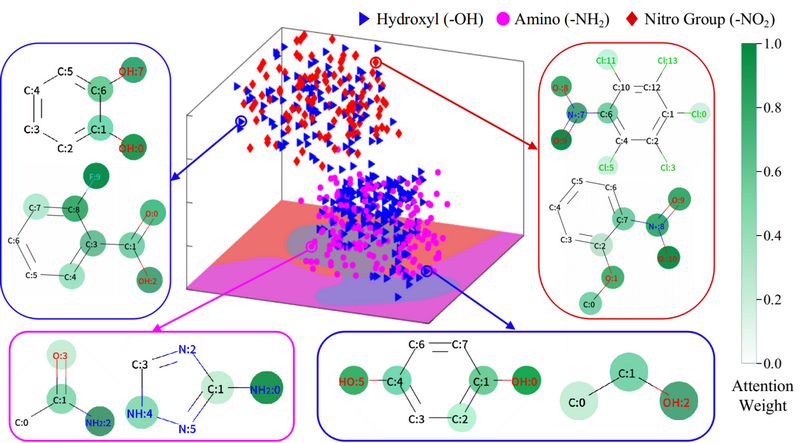
Fig 4. The t-SNE reduces the high-dimensional context vectors of the three substructures to the 3D embedding space and visualizes the attention weights learned from the Mol2Context-vec model for eight molecules.
Mol2Context-vec provides dynamic substructure representations to capture the local effects of the same substructure in different molecules. For substructures with ambiguity, the context vector generated by Mol2Context-vec correctly separates the different categories in the 3D space. In addition, the attention weights of multiple molecules to highlight that the Mol2Context-vec model can learn long-distance relationships, especially those intramolecular hydrogen bonds. The proposed model usually focuses on are very close to human’s chemical understanding of molecules.
The team of Prof. Calvin Yu-Chian Chen has long been committed to the cross research of artificial intelligence. The above research was supported by the National Natural Science Foundation of China (No.62176272), and was completed by Qiujie Lv, a doctoral student in the the School of Intelligent Systems Engineering, Sun Yat-sen University, under the guidance of Prof. Chen. Qiujie Lv is the first author of the paper, and Prof. Calvin Yu-Chian Chen is the corresponding author of the paper.
Link to the article: https://academic.oup.com/bib/article/22/6/bbab317/6357185