Progress in Precision Diagnosis and Treatment of Thyroid Cancer by AI from Professor Haipeng Xiao’s Team
Source: The First Affiliated Hospital
Edited by: Tan Rongyu, Wang Dongmei
On March 22nd, 2021, the study from Professor Haipeng Xiao’s Team “Deep learning-based artificial intelligence model assists in thyroid nodule management: a multi-center, diagnostic study” was published on
The Lancet Digital Health, the family-journal of
The Lancet focused on artificial intelligence.
Thyroid nodules are found in up to 40-66% of adults in the general population. Thyroid ultrasound is the preferred noninvasive method to differentiate malignant from benign nodules, whose misdiagnosis rate is still up to 15%-20%. Hence, Professor Haipeng Xiao’s Team committed to use deep learning technology to develop an AI diagnostic model (ThyNet), based on nearly 20,000 ultrasound images of thyroid nodules. Then the diagnostic performance of ThyNet was compared with that of 12 radiologists and the ThyNet assisted strategy was verified on the data sets from 7 centers. The accuracy of the ThyNet-assisted strategy in external multi-center verification had exceeded the experts with more than 10 years of experience in thyroid ultrasound examination. The AI-assisted strategy combining with the ACR TI-RADS guideline could reduce the proportion of patients who required invasive thyroid fine needle aspiration from 87.7% to 53.4%, while the misdiagnosis rate of thyroid cancer only increased by 0.4%.
At present, there are still controversies about how to implement AI into clinical practice and its ethical risks. This study found that half of the radiologists revised their diagnosis when their diagnosis was inconsistent with AI recommendations, while a quarter of the revised diagnosis was confirmed as an incorrect revision by pathology. This study firstly provided data on how AI affected clinical decision-making and medical behavior, and its possible ethical risks.
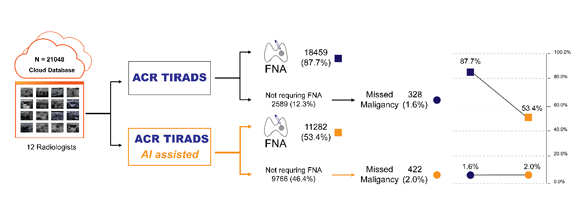
The AI-assisted strategy combining with the ACR TI-RADS guideline
The first authors of this article were Professor Sui Peng, Dr. Yihao Liu, Professor Weiming Lv, Professor Longzhong Liu and Dr. Qian Zhou. The last corresponding author of the article is Professor Haipeng Xiao from the Department of Endocrinology, the First Affiliated Hospital of Sun Yat-sen University. And the co-corresponding authors were Professor Wei Wang from the Department of Ultrasound Medicine, the First Affiliated Hospital of Sun Yat-sen University and Professor Erik K Alexander from Harvard University.
This research was led by the First Affiliated Hospital of Sun Yat-sen University, Guangzhou, China (the Clinical Trial Unit, the Department of Endocrinology, the Department of Ultrasound Medicine, the Department of Thoracic and Breast Surgery and the Medical Big Data Center). Professor Haipeng Xiao’s Team cooperated with 6 tertiary hospitals in South China (Sun Yat-sen University Cancer Center, Guangzhou, China; the First Affiliated Hospital of Guangzhou University of Chinese Medicine, Guangzhou, China; the Sixth Affiliated Hospital of Sun Yat-sen University, Guangzhou, China; the Guangzhou Army General Hospital, Guangzhou, China; the Third Affiliated Hospital of Sun Yat-sen University, Guangzhou, China; and the First Affiliated Hospital of Guangxi Medical University, Nanning, China) and Brigham and Women’s Hospital. Tsinghua University and the team of Xiaobai Century assisted in the construction of deep learning network.
This achievement of this project fully embodied the multidisciplinary, interdisciplinary and multicenter collaborative innovation and complementary advantages, and has been promoted and recommended on the homepage of
The Lancet Digital Health.
Link:
https://www.thelancet.com/journals/landig/article/PIIS2589-7500(21)00041-8/fulltext